By Assaf Feldman, CTO and co-founder, Riskified
The year 2021 did not end like we thought it would. Supply chain issues, a security breach riddling some of the world’s most popular applications and services, and thousands of flight cancellations in the face of another COVID variant have made many wonder, what’s next? If the past two years have taught us anything, it is that the retail industry and the services that support it, must be adaptive, agile, and data-centric to remain resilient in this complex environment. After all, one of the constants amidst all this change is a growing demand among consumers for security, convenience, and personalization in their shopping experience.
Consumer confidence in eCommerce is non-negotiable. It’s one of the biggest lessons we’ve learned over the past two years. Just as the pandemic has accelerated digital transformation, it too has created an environment for fraud to thrive – putting trust and security at the forefront of consumers’ minds. The good news is that thanks to machine learning, we’ve also gained insights to help retailers stay a step ahead of fraud and deliver frictionless shopping in the new year.
First and always, protect your customers’ accounts
When credentials are compromised in security breaches, one very lucrative avenue for bad actors to monetize the stolen data is to use them to access customers’ eCommerce accounts – once inside, they can order goods, use up loyalty points or steal personal identifiable information. The fallout from a data breach can linger for a long time. It can take weeks or months for stolen credentials to work their way through the process of being tested by bots, sold on the dark web, and ultimately used by human fraudsters to enter a store account and extract value. The barrier to conducting an Account Takeover Attack (ATO) is also extremely low – an amateur fraudster can buy cracked credentials on the dark web for a few dollars and gain access to an eCommerce account within minutes.
In 2021, Riskified’s systems recognized that roughly 1 in 140 login attempts had been an ATO attempt, and it is likely that a surge of credential stuffing attacks and ATOs will take place in the first few months of 2022. ATOs are a major setback for merchants. Riskified estimates that 10-25% of customers who have their store accounts taken over will never return to a merchant to shop again. While traditional bot prevention tools are able to mitigate some ATO-related risk, sophisticated credential stuffing operations can easily bypass them. These bot solutions also do nothing to stop ATO attacks conducted by humans. The most effective way to stop these attacks is to use a solution that scrutinizes a shopper’s legitimacy at the point of login.
Here is where machine learning outshines legacy fraud prevention solutions. The fundamental challenge to verifying a login is that it provides less information than checkout, where merchants fraud management typically sits. Traditional two-factor authentication also risks customer drop-off by adding friction to what is supposed to be an easy process. A machine learning platform can solve both problems by obtaining and analyzing as many data points as possible, including but not limited to IP geolocation, behavioral analytics, and spoofing detection. It can also make an accurate decision about a shopper’s identity in a matter of seconds without inconveniencing customers, who will only demand more flexibility from that point on.
Mitigate risk in payment options
One of the ways consumers expect flexibility is the payment process. While credit cards remain the dominant payment method for retail transactions, consumers continue to embrace other options. According to Capgemini’s World Payments Report, non-cash transactions will rise, with instant payments, e-money, and next-gen payment methods − such as Buy Now Pay Later (BNPL) − driving the growth. The report found that nearly 45% of consumers in 2021 frequently used mobile wallets to make payments, up from 23% in 2020. It also predicted that global B2B non-cash transactions will increase to reach nearly 200 billion transactions by 2025, up from 121.5 billion in 2020.
Merchants who cater to their customers’ preference for multiple payment choices stand to increase their conversion rate and customer satisfaction. To securely offer those choices, they must pay attention to the fact that risk patterns associated with non-credit card payments vary. In March and April 2021, Riskified data showed an upward trend in fraud levels in BNPL and direct payments, but a marked decrease in risk the rest of the year. Meanwhile, fraud rates for gift cards fluctuated throughout the year, becoming one of the riskiest payment methods during March before dropping sharply in risk levels during May. The exception was eWallets, which became steadily riskier – fraud levels increased by almost 50% since January 2021.
Adapting to rapidly changing fraud trends is difficult for legacy rules-based solutions or manual review. By contrast, machine learning solutions are trained to keep up with fraudsters’ behavior and can automatically adjust their risk thresholds according to developing trends. This ensures that merchants can broaden their payment offerings without compromising security or efficiency.
Give customers what they want: secure, flexible fulfillment
Expanding payment options is key to fulfilling customer demand for omnichannel shopping experiences, such as buying online and picking up in store (BOPIS). According to Deloitte’s 2022 Global Marketing Trends Report, companies are responding to this increased demand for hybrid experiences: three-quarters of global executives surveyed say they will invest more in creating these experiences over the next 12 months.
In-store fulfillment methods naturally carry a heightened risk. When orders are placed through a mobile device, the impermanent IP address of the mobile device and the lack of shipping address order means merchants have less data to corroborate a shopper’s identity. It’s no wonder that BOPIS orders are a fraudster’s favorite. During the crush of holiday shopping, for instance, retailers may not have time to properly verify the shopper’s identity during pickup, and no merchant wants to add unnecessary friction by subjecting customers to a drawn-out verification process.
This is where advances in machine learning can help by ensuring accurate identity verification early in the purchase process. If the AI solution already determined that a shopper was legitimate when they logged onto the merchant’s app, the more confident merchants can be at approving an eventual order. Using an AI to invisibly make a decision also means that in-store staff have less pressure on being the final point of verification.
According to Juniper Research, businesses spent $9.4 billion on digital identity verification in 2021, and that number will reach $16.7 billion in 2026. It noted that as demand grows for processes that are both low friction and high security, so will the use of AI for behavioral analytics. Fraud prevention is not just a security measure but a significant factor in the customer experience, one that needs to keep up with consumer demands for constant innovation. By embracing a data-informed, adaptive approach to fraud, merchants can ensure that they will not only keep up but will be well-prepared no matter what the future holds.
About the author
Assaf Feldman is the CTO and co-founder of Riskified. He leads the Product Development and Technology teams. Under his leadership, Riskified has built an eCommerce risk management platform with proprietary machine learning models that drive an automated decisioning engine supporting its eCommerce risk mitigation suite of products.
Assaf brings two decades of experience developing robust systems with ML algorithms and intelligent UIs for risk management applications. He was selected to be a research scientist for the Ambient Intelligence group at MIT’s Media Lab.
Related Articles
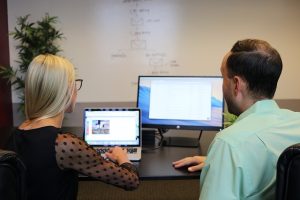
The Rise of Digital Experience Platforms (DXPs) in Software Development
Software development is evolving, as Digital Experience Platforms enable the delivery of a personalised digital cross-channel experience. A DXP delivers integrated content, Artificial Intelligence, and low-code rapid application development tools. It streamlines the work of developers as well as marketers.
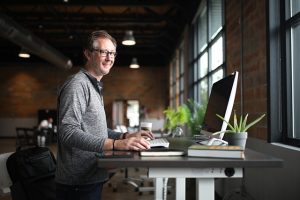
7 Employee Benefits That Can Help Your Business Increase Staff Retention
With so many businesses vying for the attention of skilled job candidates, it’s becoming more important to look for ways to make your offerings more appealing by adding unique perks that not every employer does.
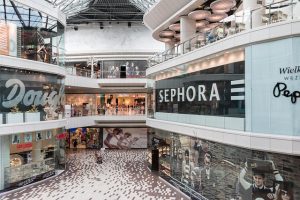
How Retailers Can Prepare for the Summer 2025 Shift
Rather than focusing on isolated touchpoints or departmental KPIs, journey management provides a way to understand where the most critical pain points lie and how to resolve them systematically across the business.
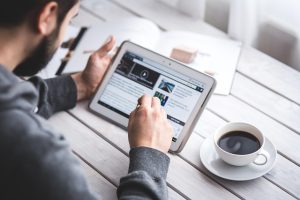
Retailers Are Drowning in Data – Journey Management Could Be the Lifeline
Rather than focusing on isolated touchpoints or departmental KPIs, journey management provides a way to understand where the most critical pain points lie and how to resolve them systematically across the business.